Slow Adoption of AI in the Semiconductor Industry
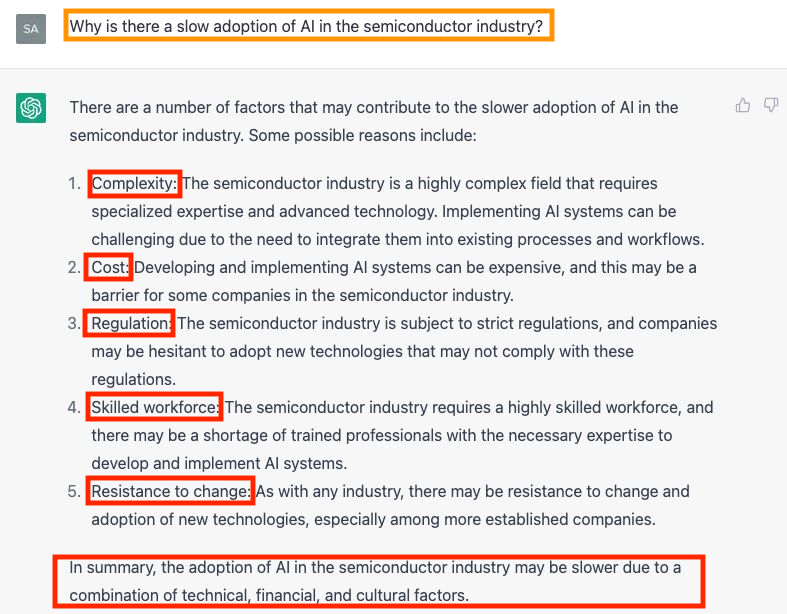
The semiconductor community is slow to adopt AI.
With the recent advances in AI, it could be irresponsible to ignore the benefits that AI can bring to the semiconductor industry.
While AI chips are touted for their advantages, there should be more focus on implementing AI within the semiconductor industry rather than just pointing to external use cases.
Some critics dismiss AI in semiconductors, citing failed internal projects or claiming it is not precise enough for design and manufacturing needs. However, the semiconductor industry must invest in AI research, development, and training across all processes to gain a significant competitive advantage.
The passing of the CHIPS and Science ACT of 2022 by the US government offers a significant opportunity for the semiconductor industry to invest in AI and secure a long-term competitive advantage. AI innovations are advancing rapidly across various industries, automating workflows, improving efficiency, and reducing time-to-market.
At VerifAI, we see considerable potential for AI in the semiconductor industry, ranging from semiconductor design to manufacturing, packaging, assembly, and testing. Empowering domain experts with AI and AI practitioners with domain knowledge will accelerate innovation, fostering disruptive growth and long-term technical resilience within the industry.
The following sections provide examples of AI's success in other industries and highlight specific use cases for AI in the semiconductor industry.
AI/ML for various Industry Segments
Various industries have embraced AI initiatives, like 'AI for Medicine,' 'AI for Manufacturing,' and 'AI for Security,' with support from both large and small corporations. These efforts aim to advance AI across entire verticals within their respective industries and involve multiple stakeholders, including private companies, government bodies, and educational institutions.
Educational institutions are actively designing specialized courses tailored to these industry segments. For example, online and offline classes from renowned platforms like Coursera, Udacity, MIT, Stanford, and UCB offer dedicated curriculums such as 'AI for Medicine' and 'AI for Healthcare,' aligning with industry needs and cultivating the next-generation workforce.
These industry-specific curriculums typically span short durations, ranging from 3 to 12 months, and focus sharply on AI/ML research or applications for the targeted industry segment. Consequently, these online AI courses have gained significant popularity among aspiring and experienced professionals, often catalyzing job growth, mobility, and career changes.
Furthermore, high school students are interested in majors incorporating AI courses into their core curriculum. As the next-generation workforce, these students present an opportunity for every industry to attract and establish a pipeline for future talent.
The figure below illustrates an example of a structured online AI course tailored for the Healthcare industry, highlighting its readiness to embrace AI for the upcoming decade. Many other industries are also following similar initiatives in their respective domains.
Figure 2: Illustrative Example of AI in HealthCare Courses
The semiconductor industry needs initiatives such as the one highlighted above.
AI/ML has been Transformational for many Industries
Machine Learning / AI has revolutionized numerous industries beyond what could have been imagined just five years ago. Notably, even non-domain experts have achieved remarkable outcomes by leveraging AI to tackle some of the most challenging technical problems in the scientific realm.
Below are a few examples of complex scientific problems that have benefited from AI.
Protein Folding:
Google's DeepMind, an AI company, has made significant strides in protein synthesis and folding. Their AI algorithm, AlphaFold, has successfully predicted the 3D structures of nearly all known proteins based solely on their 1D amino acid sequences.
With the ability to predict 3D structures for over 200 million proteins, AlphaFold has outperformed traditional methods domain experts use.
This groundbreaking innovation will accelerate new drug discoveries by several decades. Instances where non-domain experts surpass domain experts using AI are still uncommon, but the potential for such achievements is increasing rapidly due to the rapid advances in AI capabilities.
Digital Pathology and Radiology
AI is revolutionizing digital pathology and Radiology in healthcare by aiding pathologists in enhancing prognosis, diagnosis, and disease prediction. Pathologists can achieve faster and more cost-effective diagnoses and predictions through advanced AI image analysis.
As digital pathology becomes more efficient with AI integration, the demand for medical doctors in pathology may decrease. Instead, there will be an increased need for skilled nurse practitioners and lab technicians with specific AI training for digital pathology.
The rise of automation and AI in the medical field may lead to the displacement of specific jobs, but it will also create new roles that do not currently exist. Future medical jobs may require less traditional education, with a greater demand for workers trained in specific skill sets, like AI training for digital pathology.
While the demand for pathologists is projected to grow modestly by 3 percent from 2020 to 2030, other related medical fields may experience higher growth rates. Embracing AI in digital pathology can help prepare the workforce for the evolving landscape and ensure a well-trained talent pool with targeted skill sets.
Industrial Robotics
Industrial Robotics hardware is used in various factories and warehouses for over two decades. These robots have typically been single-application units programmed for specific tasks.
However, recent advancements in AI algorithms, particularly in Reinforcement Learning and Computer Vision, have opened up new possibilities. Industrial robots can now leverage AI to learn and perform multiple functions through observation without explicit pre-programming.
This breakthrough has the potential to revolutionize industrial robotic applications, leading to a strong focus on AI research to develop multi-functional robots capable of learning through observation and trial and error.
AI's transformative impact extends beyond industrial robotics, permeating numerous industries and underpinning technologies used in both industrial and consumer applications.
Research and Application Areas for AI in Semiconductors
Semiconductors encompass various fields, from computer architecture and design to physical manufacturing, packaging, assembly, and testing. However, despite significant research opportunities, we observe infrequent sparks of AI innovation in this industry from various companies.
AI holds immense potential in each stage of the semiconductor process, offering opportunities to enhance efficiency, accuracy, and time-to-market. Implementing AI algorithms and models at each step can lead to high levels of automation and automated decision-making.
The application of AI is relevant throughout the semiconductor design, development, manufacturing, and testing stages. Recent advancements in Large Language Models (LLM), Reinforcement Learning (RL), and Computer Vision (CV) provide promising avenues for exploring how these technologies can benefit semiconductor processes.
AI for Semiconductor Material Discovery, Example:
Material discovery has significant potential for the accelerated synthesis of new materials through AI. This research area is crucial because of the continuous demand for new materials driven by sustainability and toxicity reduction.
An example from Nature Computational Materials showcases AI's effectiveness in reducing the toxicity of PhotoAcid Generator (PAG) molecules, essential compounds used as photoresists in semiconductor manufacturing. Without AI, discovering and testing these new PAGs would take years. However, AI-enabled a 100-X reduction in the number of PAG candidates for analysis by a human toxicology expert.
Figure 4 - *Source: Accelerating materials discovery using artificial intelligence, high-performance computing, and robotics - www.nature.com/npjcompumats (Nature Computational Materials), April-26-2022, (Edward O. Pyzer-Knapp, Jed W. Pitera, Peter W. J. Staar, Seiji Takeda, Teodoro Laino, Daniel P. Sanders, James Sexton, John R. Smith, and Alessandro Curioni)
AI for Manufacturing, Example:
AI can substantially benefit the manufacturing process by creating AI models, known as Digital Twins, for each process and subprocess step.
Specialized machines and robots require constant supervision and maintenance in semiconductor manufacturing facilities. By capturing the behavior and attributes of these machines into digital AI models, predictive maintenance schedules, failure predictions, and simulations of different manufacturing process behaviors become possible.
These digital AI models offer significantly higher speed and accuracy than trial and error or random tuning methods on live equipment. They can effectively capture and update the behaviors of complex functions and processes throughout the manufacturing cycle.
Defect detection, wafer disposition, and sorting are time-consuming manual steps. However, with AI, these processes can be vastly improved, streamlining and enhancing their efficiency.
Figure 5 illustrates the various steps where AI can be used to improve efficiency, yield, and time-to-market.
AI for Semiconductor Architecture and Design, Example:
AI is revolutionizing Hardware Design space exploration by seeking optimal combinations of microarchitecture, memory, and compute usage to enhance Performance, Power, and Area (PPA). Traditionally, experienced micro-architects and performance engineers handle this task, relying on their deep understanding of the design's microarchitecture. However, this process is time-consuming and resource-intensive.
Recent research demonstrates the potential of AI to automate trade-offs by learning from previous designs and selecting the best architecture to achieve the required PPA. This advancement can significantly impact semiconductor companies, as AI-recommended microarchitectures may surpass PPA requirements.
Moreover, the semiconductor industry is exploring AI for autonomous optimizers that can automatically improve PPA during Chip implementation. These efforts promise a higher level of automation and reduced time-to-market. Leveraging advanced AI techniques, like Reinforcement Learning, these initiatives mark the initial stages of autonomous chip implementation, offering considerable potential for expediting the overall process.
AI for Semiconductor Design Verification, Example:
Design Verification and Validation (DVV) is critical for ensuring that Integrated Circuits (ICs) function securely, reliably, and as intended.
Industry data highlights that the cost of a design error in an IC can amount to tens or even hundreds of millions of dollars.
DVV represents the primary bottleneck in chip design and production, consuming over 60% of the time and cost. This DVV bottleneck contributes to longer time-to-market and exacerbates supply chain challenges. Siemens' recent research underscores the high demand for Design Verification Engineers.
By leveraging AI research and applications, the semiconductor industry can accelerate DVV 100X within a decade. The speedup in DVV will drive a significant boost in semiconductor innovation, alleviate supply chain issues, and safeguard US Economic competitiveness and security
Figure 6 - Source: Part 8: The 2022 Wilson Research Group Functional Verification Study, By Harry Foster • December 12, 2022)
Figure 7 - Source: Part 8: The 2022 Wilson Research Group Functional Verification Study, By Harry Foster • December 12, 2022)
Various design verification stages offer ample opportunities to implement AI solutions that dramatically speed up DVV. Embracing AI in these processes can lead to transformative advancements in the semiconductor industry.
AI for Semiconductor Testing and Validation, Example:
Product testing and validation involve the testing and validation of post-silicon wafers and assembled parts, a time-consuming process often causing delays in shipping.
AI offers the potential to significantly accelerate post-silicon Test and Validation, achieving up to a 100X increase by automating test and stimulus generation, facilitating debugging, and quickly identifying bugs.
This domain presents numerous research areas and substantial opportunities for leveraging AI techniques to expedite post-silicon test and validation processes dramatically.
AI for Semiconductor Hardware Security, Example:
Protecting hardware from malicious attacks is an increasingly challenging task, with bad actors utilizing advanced methods like side-channel attacks that monitor electromagnetic signals from chips to predict execution flow.
Extensive research has been done on mitigating and preventing hardware attacks, and recently, AI has been employed to model both attacker and defender behavior, leading to innovative solutions.
The field of AI for hardware security is rapidly growing, presenting significant opportunities for research and development.
Conclusion
Although AI adoption in the semiconductor industry has been slow, it holds enormous potential to drive disruptive innovation.
From material discoveries to chip design, manufacturing optimization, and post-silicon test and validation, AI can accelerate innovation, enhance productivity, and reduce time-to-market.
To fully unlock these benefits, the semiconductor industry must accelerate its investment in AI research, training, and workforce development.